The authoritarian war on due process – Daily News
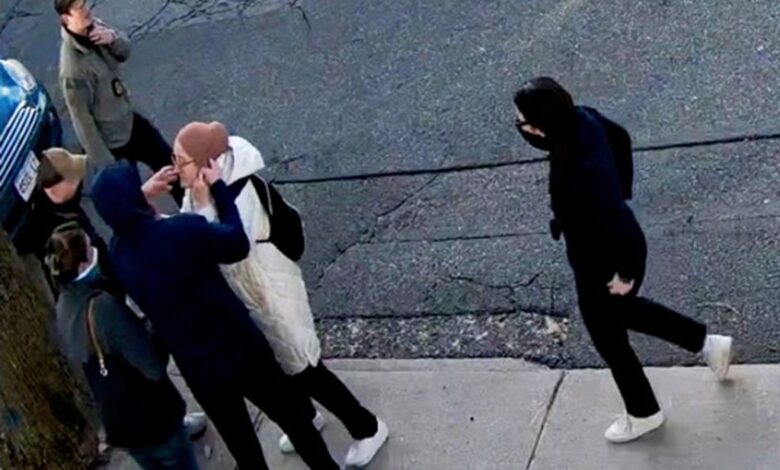
“If Trump can disappear them, he can disappear you.”—Robert Reich
The war on due process is here.
No trials. No hearings. No rights. Just indefinite detention and secret deportations.
This is the fate that awaits every one of us, not just immigrants (legal or otherwise), if the government’s war on the Constitution remains unchecked.
More than two decades after the U.S. government in its post-9/11 frenzy transported individuals, some of whom had not been charged let alone convicted of a crime, to CIA black sites (secret detention centers located outside the U.S. authorized to torture detainees) as a means of sidestepping legal protocols, the Trump Administration is using extraordinary rendition to make those on its so-called “enemies list” disappear.
The first round of arrests and deportations to a mega-prison in El Salvador supposedly targeted members of the infamous Venezuelan gang Tren de Aragua.
Carried out with little evidence and without court hearings or due process, these roundups reportedly may also have swept up individuals with no apparent connection to gang activity apart from common tattoos(firearms, trains, dice, roses, tigers and jaguars) and other circumstantial evidence.
In a particularly Kafkaesque explanation for why some of the Venezuelan migrants who have no criminal records were targeted for arrest and deportation, government lawyers argued in court that their lack of a criminal record is in itself cause for concern.
In other words, the government is prepared to preemptively arrest and make people disappear, without any regard for legal protocols or due process, based solely on the president’s claim that they could at some point in the future pose a threat to national security.
This takes pre-crime and preemptive arrests to a whole new sinister level of potential abuses.
Are you starting to sense how quickly this could go off the rails?
This is how democracies collapse. This is how rights disappear overnight.
As lawyers challenging the government’s overreach warned, “If the President can designate any group as enemy aliens under the Act, and that designation is unreviewable, then there is no limit on who can be sent to a Salvadoran prison, or any limit on how long they will remain there.”
Also among those in danger of being made to disappear without any legal record or due process are individuals who have not been charged with or convicted of any crimes.
The most egregious of these incidents involve college students, scientists and doctors, all of them legal permanent residents of the U.S. who, while never having been charged with a crime, are accused of threatening national security by taking part in anti-war protests over the growing death toll in Gaza as a result of the Israeli-Hamas war, or sympathizing with the Palestinians, or being associated with someone who might sympathize with the Palestinians.
When merely exercising one’s right to criticize the government in word, deed or thought is equated to an act of domestic terrorism, we are all in trouble.
The mass arrests and roundups thus far have been so haphazard that there is a very real likelihood that innocent individuals have also been swept up and deported.
American citizens could very well be next in line for this kind of treatment.
This is the danger of allowing any president to use expansive wartime powers to bypass the Constitution’s prohibitions against government overreach and abuse: suddenly, everything that challenges the government’s authority becomes a national security threat and every dispute a national emergency.
Through his use of executive orders, proclamations and so-called national emergencies, President Trump has essentially declared war on the rule of law.
Make no mistake: while immigrants, illegal and legal alike, have largely been the first victims of the Trump administration’s efforts to circumvent the Constitution in order to make them disappear, it’s our very freedoms that are being made to disappear.
At the heart of these freedoms is the right of habeas corpus.
Translated as “you should have the body,” habeas corpus requires the government to either charge a person or let him go free.
While the Constitution allows the writ of habeas corpus to be suspended in cases of rebellion or invasion when public safety is imperiled, the Trump Administration’s efforts to keep the nation in a permanent state of emergency in order to justify its power grabs leaves “we the people” subject to the kinds of arbitrary mass round-ups, arrests and deportations that have been favored by despots and dictators.
This is usually where the self-righteous defenders of Trump’s blatantly unconstitutional tactics insist that the protections of the Constitution only apply to U.S. citizens.
They are wrong.
At a minimum, as the U.S. Supreme Court has affirmed, the rights enshrined in the first ten amendments to the Constitution apply to all people in the United States, regardless of their citizenship or immigration status. Those rights include free speech, peaceful protest and criticism of the government, assembly, religious freedom, equal protection under the law, due process, legal representation, privacy, among others.
Then again, what good are rights if the government doesn’t respect them?
What good are rights if the president is empowered to nullify them whenever he wants?
For that matter, what good is a government that betrays its own citizens?
History has shown us that when governments operate without checks and balances, tyranny follows. The question is not whether mass arrests and indefinite detentions could be expanded to American citizens—it’s how long before they are.
If we allow the erosion of due process, if we accept that a president can unilaterally decide who is a threat without oversight, then we have already lost the freedoms that define us as a nation.
We must demand accountability. We must challenge policies that violate constitutional protections. We must support organizations fighting for civil liberties, educate ourselves on our rights, and refuse to be silenced by fear. Because when the government starts making people disappear, the only way to stop it is by making our voices impossible to ignore.
As I make clear in my book Battlefield America: The War on the American People and in its fictional counterpart The Erik Blair Diaries, freedom does not die in a single act of repression—it dies when the people surrender their rights in exchange for false security.
The Constitution can’t protect us if we don’t protect it.
John W. Whitehead is founder and president of The Rutherford Institute. Nisha Whitehead is the Executive Director of The Rutherford Institute. Information about The Rutherford Institute is available at www.rutherford.org.