AI is helping scientists decode previously inscrutable proteins
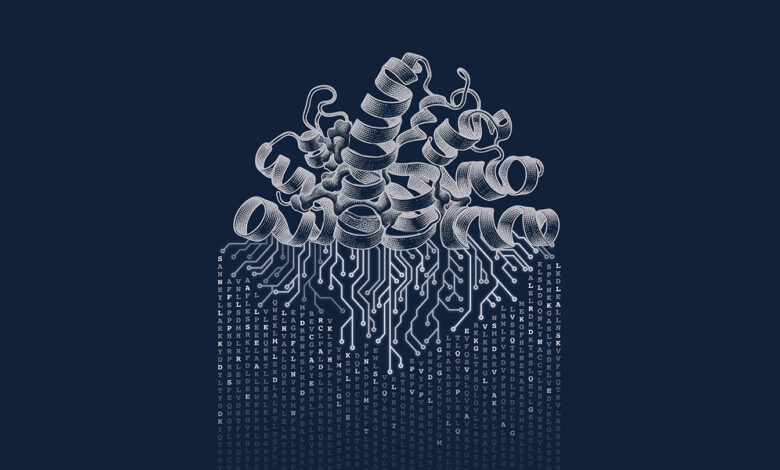

Generative artificial intelligence has entered a new frontier of fundamental biology: helping scientists to better understand proteins, the workhorses of living cells.
Scientists have developed two new AI tools to decipher proteins often missed by existing detection methods, researchers report March 31 in Nature Machine Intelligence. Uncovering these unknown proteins in all types of biological samples could be key to creating better cancer treatments, improving doctors’ understanding of diseases, and discovering mechanisms behind unexplained animal abilities.
If DNA represents an organism’s master plan, then proteins are the final build, encapsulating what cells actually make and do. Deviations from the DNA blueprint for making proteins are common: Proteins might undergo alterations or cuts post-production, and there are many instances where something goes awry in the pipeline, leading to proteins that differ from the initial genetic schematic. These unexpected, “hidden” proteins have been historically difficult for scientists to identify and analyze. That’s where the machine learning tools come in.
The AI models, called InstaNovo and InstaNovo+, are a step toward “the holy grail” of protein research: to unravel the genetic identity of previously unstudied proteins en masse, says Benjamin Neely, a chemist and protein scientist at the National Institute of Standards and Technology in Gaithersburg, Md.
With continued advances and testing, these tools or similar ones are “going to be powerful. It’s going to let me see things that I can’t normally see,” says Neely, who was not involved in the study. Many non-model organisms haven’t been well studied, and their proteins are poorly cataloged. As a hypothetical, Neely suggests the new tools could be used to find the obscure kidney proteins that allow stingrays to move between brackish water and the ocean.
AI has already transformed how researchers predict protein folding with a tool called AlphaFold. And machine learning–powered protein design earned a Nobel Prize in 2024. Filling long-standing gaps in protein sequencing is poised to be the next AI leap in the field, Neely suggests.
InstaNovo (IN) is structured similarly to OpenAI’s GPT-4 transformer model and trained to translate the peaks and valleys of a protein’s “fingerprint,” plotted through mass spectroscopy, into a string of likely amino acids. These amino acid sequences can then be used to reconstruct and identify the hidden protein. Instanovo+ (IN+) is a diffusion model that works more like an AI image generator and is primed to take the same initial information and progressively remove noise to produce a clear protein picture.
IN and IN+ are not the first attempts to apply machine learning to protein sequencing. But the new study demonstrates how far the technology has come in recent years — edging ever closer to real-world utility, largely thanks to expanding protein analysis databases like Proteome Tools, which can be used to train AI models. These were the data used to develop and train IN and IN+, but the models’ analyses extend beyond the proteins in existing databases. They can suggest possible protein segments that haven’t yet been cataloged.
Both tools individually show promise across a spate of tests compared with results from a previously released AI transformer protein decoder called Casanovo and from the database search method most commonly used to ID unknown proteins. In straightforward protein sequencing tests, the models don’t outperform database search, yet they seem to excel in more complicated trials.
One especially challenging task is sequencing human immune proteins, which are uniquely tough to analyze with standard methods because of their small size and amino acid composition. The researchers report that IN finds about three times as many candidate protein segments as classic database searching, going from about 10,000 identified peptides to more than 35,000. And IN+ finds about six times more. Used together, the models’ combined performance offers an even larger boost.
Based on the thorough validation presented in the study, Amanda Smythers, who specializes in protein analysis, says she’d be eager to try the tools. A chemist at Dana-Farber Cancer Institute in Boston, Smythers imagines using the AI models to answer questions like why pancreatic cancer commonly triggers rapid muscle wasting and fatigue. Proteins made by cancer cells or disruption of normal protein function in noncancer cells could be at fault. “It’s a really important piece of biology that we don’t understand yet,“ Smythers says.
Bringing obscure protein sequences to the surface (whether they’re from cancer cells or stingray kidneys) could enable the possibility of neutralizing harmful ones or harnessing beneficial ones to treat disease.
Still, the new models have limitations.
The possibility of false positives, which the study authors estimate at around 5 percent, means the AI outputs require extra verification, says coauthor Konstantinos Kalogeropoulos, a computational bioengineer at the Technical University of Denmark in Lyngby. And how to best evaluate these AI tools remains an open question, notes William Noble, a developer of Casanovo and a computer scientist and proteomics researcher at the University of Washington in Seattle.
Finally, AI sequencing is not a replacement for database searching, Smythers says. It’s a supplement. “There’s never one single tool that’s good for every job,” she says. “However, it’s tools like this that really help us keep progressing the field further.”